Summary
“Data lineage is defined as a data life cycle that includes the data’s origins and where and how it moves over time.”
Data Lineage (DL) has been promised, conceptualized and even implemented in many organizations to be the holy grail of understanding data within complex technologies.
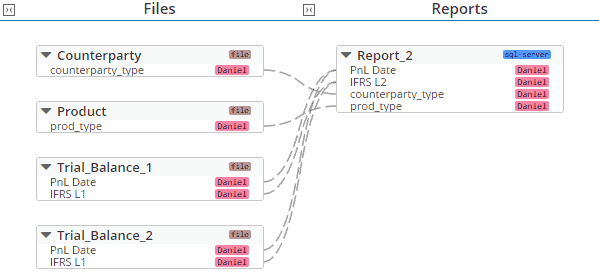
Data lineage in financial services
Data lineage gained prominence in the Financial Services from the regulatory framework of the FED (CCAR etc.) and ECB (BCBS 2329). With regulators asking banks for the origin of data in submissions, Data Lineage is a convenient form to show the origins and paths of data from source systems to Y9C form for example.
Myth of data lineage
DL has been touted to be many things it is not:
· Evidence of data integrity for regulators (arguably yes).
· Technology teams can look at the data flows to shorten the requirement phase of any new project (Not in most cases)
· Data Quality teams can use DL to create and manage quality problems in production systems (hardly)
· A convenient way for Business Analysts to source their reporting data (never seen it work).
Types of data lineage
Firms have adopted different methods to demonstrate DL in their enterprise. Some common examples (in reverse-order of complexity):
DL1) Logical Data Lineage
Functional or Role based Data mapping
DL2) System Data Lineage
Power Designer based data models or System-level flows
DL3) Attribute Data Lineage
Attribute-level mapping of transformations and data flows
Challenges in implementing data lineage
Complexity of representing data flows is of course directly proportional to the number of systems, databases and business reporting/applications. In large banks or insurance companies, the legacy technology debt accrued over the years makes this task difficult (if not impossible).
Lack of documentations, data models makes the process to decipher and build data flow diagrams is a frequent complaint. Most system-level and attribute-level lineage results are either grossly incorrect or such little coverage to be useful.
Practical considerations
DL1: A good place to start is via organization-wide surveys or interviews with business users, SMEs and Technology teams. This logical mapping is usually a very good start to get a feel for the complexity involved in deciding whether to pursue with more detailed data lineage exercise. [Technology: Excel, Vizio, PowerDesigner, Erwin etc.]
DL2: This is generally a technology-led exercise that only has the expertise to document these flows. [Technology: Excel, Vizio, PowerDesigner, Collibra etc.]
DL3: The presence of data transformation layers (ETLs) adds a level of complexity that cannot be solved by superficial hand-waving. If ETL logic cannot be touched as part of the lineage exercise, DL3-level Lineage exercises will fail. [Technology Tools: Vizio, Graph Databases such as neo4j, Collibra, EA tools etc.]